About the Project
Tobacco marketing, restricted almost exclusively to the point-of-sale in recent years, is extremely effective in getting more people to consume and fewer to quit these deadly products. The lack of empirical documentation linking product exposure to behavior, however, is a key obstacle to the adoption of additional restrictions on point-of-sale tobacco advertising. The goal of this project is to map point-of-sale tobacco marketing practices across New York City using automated detection of tobacco signage in street-level imaging data. We propose to build and train convolutional neural networks, which are particularly effective at detecting objects in images, to identify and classify outdoor advertisements of cigarettes and smokeless tobacco. Previous analyses of visual data in public health research involving manual image coding, though made more efficient over time with the help of crowdsourcing, are prohibitively costly and time-consuming. The importance and motivation of the project stems from the immediate and comprehensive effect of tobacco advertisements on its sales and consequently on public health. Once the model is developed, it can be used in measuring exposure of at-risk communities to tobacco displays.
Problem Statement
The gap between how humans are able to perceive the world, both graphically and semantically, and how computers can be trained to automatically interpret imagery data, is rapidly closing. The automated tobacco signage detection model employed in this project involves Faster R-CNN, which is the state-of-the-art convolutional neural network related to image recognition. By efficiently discriminating the background and target within an image, this model enables a detection algorithm to focus on the target, which are the areas that are more likely to contain tobacco signages. Development of a model that can identify signs, but also distinguish between tobacco ads and other types of signs (i.e. stop signs, cellular service provider ads), is critical to the success of the model when applied to images that the model has not seen before. In this project, we seek to improve the existing Faster R-CNN model in its ability to identify signs and discriminate tobacco signages from other types of signs in NYC.
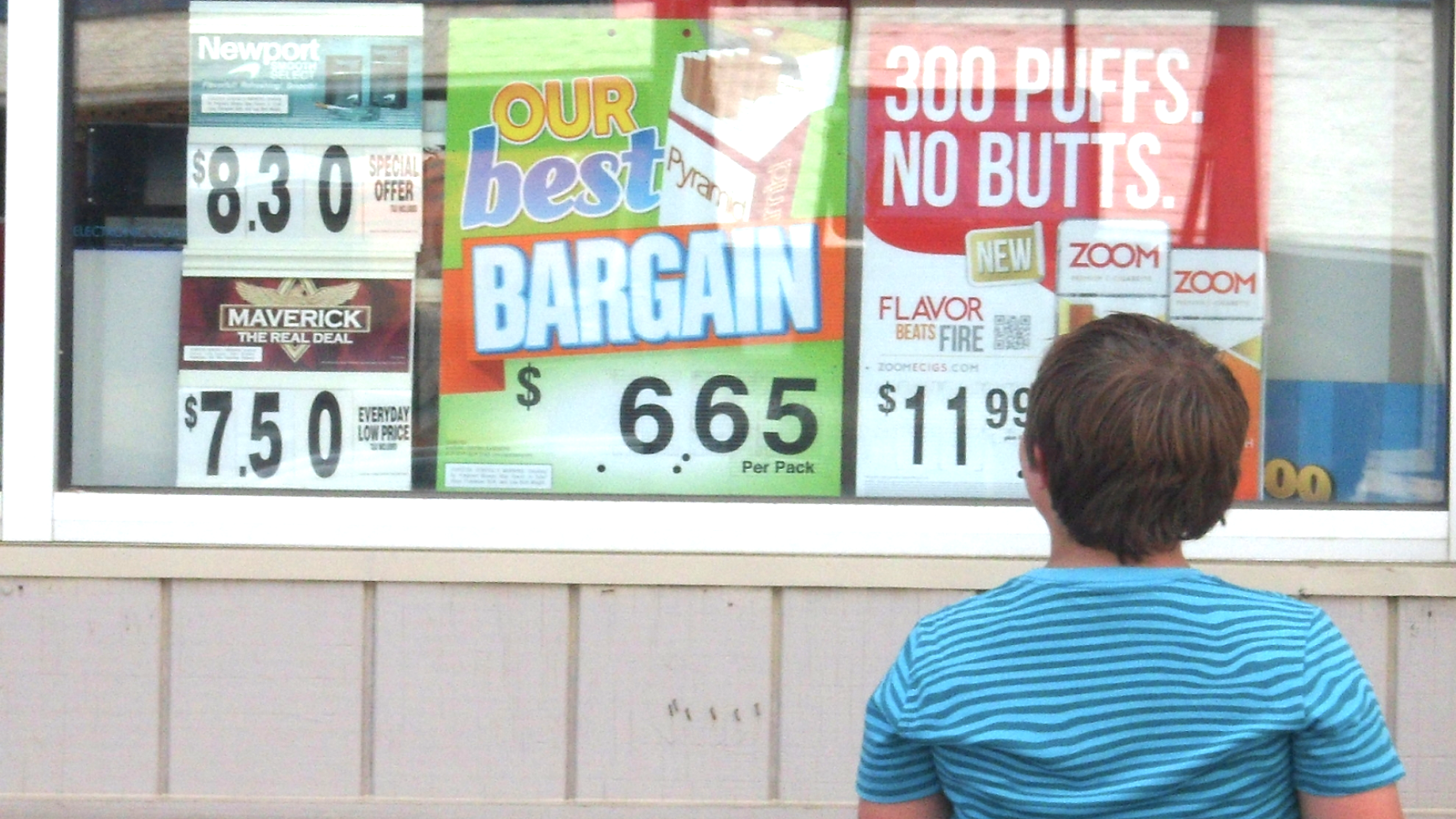